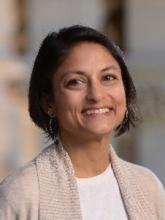
(English captions & Hindi subtitles available)
About the Seminar:
Using administrative data on over 4 million hospital visits, Radhika Jain documents striking gender disparities within a government health insurance program that entitles 46 million poor individuals to free hospital care in Rajasthan, India. Females account for only 33 percent of hospital visits among children and 43 percent among the elderly. These shares are lower for more expensive types of care, and far lower than sex differences in illness prevalence can explain. Almost two-thirds of non-childbirth program spending is on males. She combines these data with patient survey, census, and electoral data to show that 1) the program does not fully offset the costs of care-seeking, which leads to disparities in utilization because households are willing to allocate more resources to male than female health; 2) lowering costs does not reduce disparities because males benefit as much as females do; and 3) long-term exposure to village-level female leaders reduces the gender gap in utilization, but effects are modest and limited to girls and young women. In the presence of gender bias, increasing access to and subsidizing social services may increase levels of female utilization but fail to address gender inequalities without actions that specifically target females.
About the Speaker:
Radhika Jain is the Asia Health Policy Postdoctoral Fellow for 2019-2022 at the Walter H. Shorenstein Asia-Pacific Research Center (Shorenstein APARC) at Stanford University. Her research focuses on health care markets, the effectiveness of public health policy, and gender disparities in health. She completed her doctorate in the Department of Global Health at Harvard University in 2019. Her dissertation examined the extent to which government subsidies for health care under insurance are captured by private hospitals instead of being passed through to patients, and whether accountability measures can help patients claim their entitlements. Jain's research has been supported by grants from the Weiss Family Fund and the Jameel Poverty Action Lab (JPAL). She has worked on impact evaluations of health programs in India and on the implementation of HIV programs across several countries in sub-Saharan Africa. She also held a doctoral fellowship at the Center for Global Development. At Shorenstein APARC, she is starting new work on understanding the factors that contribute to poor female health outcomes and interventions to increase the effectiveness of public health insurance.
FULL TRANSCRIPT:
Nafiz Hassan:
So hello and welcome to the fifth event in fall seminar series. My name is Nafiz Hassan. I'm a postdoctoral research scholar at CASI and along with my colleagues moderate the series. The seminar, some of you already know comprises talks by diverse range of scholars from India and the US and a variety of critical and contemporary topics. As speakers come from a wide range of disciplines, including political science, anthropology, sociology, economics, and public policy, we have these talks typically on Thursdays at noon, Eastern time. Today's a bit of an exception, so please sign up for them on the CASI website. So before I introduce today's speaker, just to put in a plug for next Thursday, that is November 4th, we have Dr. Paul Novosad from Dartmouth College presenting his work on In-group Bias in the Indian judiciary, which evidence from 5 million criminal cases. So please register for that on our website if you're interested.
So without further ado, I'm delighted to welcome Dr. Radhika Jain to the seminar today. Dr. Jain is the Asia health policy postdoctoral fellow at Stanford University and an affiliate of the Stanford King Center. She completed her doctorate in the department of global studies at Harvard University in 2019. Her research focuses on healthcare markets, the effectiveness of public health policy and social economic disparities in health. Prior to her doctorate, she worked on impact evaluations of health programs in India, and on the implementation of HIV programs in sub-Saharan Africa. About today's talk. Using administrative data and over 4 million hospital visits, Dr. Jain documents striking gender disparities within the government health insurance program that entitles 46 million poor individuals to free hospital care in Rajasthan, India. Females account only 33% of hospital visits among children and 43% among the elderly. These shares are lower for more expensive types of care and far lower than sex differences than illness prevalence can explain.
Almost two thirds of non childbirth program spending is on males. She combines these data with patient survey census and electoral data to show that first the program does not fully offset the costs of care seeking, which leads to disparities in utilization, because households are willing to allocate more resources to male than female health. Second, lowering costs does not reduce disparities because males benefit as much as females do. And third, long-term exposure to village level female leaders reduces the gender gap and utilization, but effects are modest and limited to girls and young women. In the presence of gender bias, increasing access to and subsidizing social services may increase levels of females utilization, but fail to address gender inequalities without actions that specifically target females. So before I turn it to our speaker, just to remind you, we're going to have a similar format like last time.
So if you have questions at the end, could you please use the chat box to send them directly to me, Nafiz Hassan, and I will call on you to pose your question to our presenter. Please keep your question brief and to the point so we can get to as many as possible and apologies in advance, if I can't get to everyone. Also, please use the chat box only for questions. Finally, please be mindful about muting your mikes throughout the duration of the event. And also please remember that you cannot record this presentation, without prior permission from the presenter. Once again, thank you for your interest and for being here today. With that, I'm going to turn to Dr. Jain to take us away.
Radhika Jain:
Thanks so much for the introduction Nafiz, and to everybody at CASI for inviting me. I'm excited about this talk. I'm going to talk about gender disparities in government health insurance in India today. And this is from a joint research paper with Pascaline Dupas who's a professor of economics at Stanford University. And I should say, this is a working papers so if you have thoughts and comments, they'd be welcome. But also I have about 30 minutes to speak today. So I'm going to give you a broad overview, but you can find the more detailed paper online on the website. So India is consistently ranked among the bottom five countries in the world for female health and survival. And there's a large literature now that many people here will be familiar with documenting substantial gender bias in healthcare inputs, whether that's immunization care seeking of the doctor, et cetera, and shows that this leads to substantially worse female health outcomes. And the welfare impacts of this are substantial, are serious, right?
So it's now been estimated that about 63 million missing women in India and a less known fact actually is that the majority of these are not missing at birth. This is not from selective abortion. In fact, they're not even just, the majority is not even childhood. A lot of these are women that are missing in adulthood. Essentially women are not living as long as they should in India. And the fact that we've seen this in adulthood sort of further suggests that, just varies in inputs along the life cycle may be playing a role. So for a long time, subsidizing health care and increasing access to health services has been universally a sort of key policy to reduce inequality. In India, historically, this has been through direct provision of healthcare through the large network of public hospitals and health centers. But since 2008, the government has also started scaling up government health insurance programs, which provide free care to poor households at all public, as well as impaneled private hospitals.
And there've been state programs all over the country. An explicit goal of these programs is ensuring universal and equitable access. And this is a major strategy, to give you the sense in 2019, the central government launched the national PM-JAY program that covers, it aims to cover the poorest 40% of the Indian population. So this is a major piece of Indian health policy. But it remains unclear, it hasn't really been studied, whether subsidizing hospital care in this context reduces gender inequalities and healthcare utilization. And that's what this paper is going to study and whether this is true and if not, why? So the program I study is the BSBY program or the Bhamashah Swasthya Bima Yojana, which was launched in December 2015 and Rajasthan. Under the program, poor households are eligible.
This is determined by state poverty lists. Households are automatic once they meet the poverty status, they are automatically enrolled and eligible. They don't pay any premium or copay. They're entitled to free coverage of about 1400 pre-specified services. And this coverage includes tests, medicines, the duration of your stay, so once you get to the hospital, you're really not supposed to pay anything for your care. As long as is eligible. And households can get kids' care up to a value of about 3.3 Lakhs or about $5000 per year annually, and impact is almost no households actually reach this gap. And they can get care at all public health facilities, which was always the case, as well as impaneled, private hospitals. And sort of at the end of our study period, I think there were about 1600 hospitals, two- thirds of which were private. And then hospitals are paid directly by the insurer for the services provided.
So that patients really face nothing, should face no costs. And this program is very similar in design, to the national PM-JAY program that I mentioned earlier and was in fact rolled into it in late 2019. Right? So I think that the many of the results we find here may sort of extrapolate to programs across the country. Just to give you a sense of the hospitals, what we mean by hospitals. Most of the hospitals, that we're looking at, these are private hospitals if you are, it's outside of [Jaipur 00:08:24]. Three to four stories, they're relatively small and they tend to be located in these sort of district headquarters towns rather than right in the villages. And, this is just comparing the delivery ward in the private hospital, on the left and the public hospital on the right. This is not universally the case, but quite often you find that equality, as you could see them sort of in the public sector, it's a little bit less sterile.
It's not clear that those sheets have been changed. So often the quality is certainly perceived to be higher in private than it is in public. Okay. So that's just sort of some background. So I think one of the things that I liked about this paper actually is the data that we put together that we spent some time doing, putting together. Through a partnership with the government, we got access to the programs, administrative data. So we have insurance claims for every hospital visit from December, 2015 when the program was launched through late 2019. And that's about 6 million hospital visits. These claims data include patient name, age, sex, phone number, and address, many entered. And then a little bit on the hospital, the name, whether it's public or private and it's sort of district location, as well as the service that was provided and the date.
And, since we rely on the recorded sex and these insurance data, we did some surveys with patients to confirm... Find that actually the sex data has about 98% accuracy. So that's good. For the purposes of this study, we exclude the first year of the program because administrative data was a blessed, good quality at that time for the most part. [inaudible 00:10:10] childbirth, because those are purely for females. And a lot of what we do has been comparing male and female utilization. And we also exclude infant claims because the information that's recorded for them is actually often the mother's information. So it doesn't tell us very much about the sex of the child. And so that leaves us with about 3.3 million observations. We then take this data. We geocode, or essentially get the GPS coordinates of all of the hospitals in the program, by running it through the Google API, and then taking the patient addresses that were recorded in the data.
We sort of cleaned them up and then link them, match them to census locations, and therefore are able to figure out the geocode the patient resident locations as well. And so what this then allows us to do is essentially calculate the travel distance, the distance to the closest hospital for every patient present location, as well as the distance actually traveled for each hospital visit, within BSBY. And we can do this for... We geocode almost all of the hospitals in the program, it was 2.3 million of all of the visits. And again, patient surveys confirmed that the accuracy of our geocoding was actually quite high and reliable. And so, and then additionally, we link these data to population censuses, as well as village level electoral histories over several decades and household surveys and you'll see how this becomes relevant down the line.
One thing to point out is that because we only have the insurance claims data, we only have what people actually did observe, we see what utilization, we don't have the lists of those actually eligible. So I can't tell you what share of women that were ineligible and enrolled, use this care or what share of me use care, use BSBY, instead what a lot of this analysis is going to focus on to study gender differences is a female share of visits. So it's kind of comparing male and female levels of utilization, as well as gender differences in care-seeking patterns.
Okay. So first part of the paper is very much descriptive, and it's simply going to document, try to document carefully, large gender disparities in healthcare utilization. So what this graph, I don't quite know what to do about that, but what this graph is showing you is the female share of BSBY visits on the y-axis by each group on the x-axis. And what you see is, the gray line is 50%, which is that if men and women were using BSBY equally, you should see the female share, be about 50%. Instead, what you see is that especially amongst children and the elderly, the female share is far lower. Women account for a far lower share of all hospital visits. Amongst children only about 33% of all hospital visits are for girls relative to boys, 70% of the boys, and amongst elderly, the female shares about 43%.
And overall it's about 45% right now. The first thing you might think is, "Well, we know that you've already told me that there are all these missing women. So the population sex ratio is in doubt so maybe that's what's driving it." So these red diamonds are the female share of the population for the population census. And then the green diamonds. I do it using also population health surveys, focusing just on the poorest part of the population in case you think those saturations are very different. And what you see is, yes, amongst children, especially the female sex ratio is lower, or the female share the population is lower, but nowhere near the levels they would need to be, to explain the gender disparities that we see in BSBY, okay.
Oops. Okay. So the next question is, well what if 50% is a wrong mark? What if women are less likely to be ill than men? And so you shouldn't see women utilizing BSB by as much as men. So to study this, what we did was we took the global burden of disease, we got from that estimates of sex-specific prevalence of illness. And from that, with a little bit of math, we can sort of back out what share of all people with a certain condition in the population are female, right? This is kind of the true illness in the population. So to make that a little clearer, and let's focus on that first panel, which is for chronic kidney disease. The red dots are the female share in the global burden of disease.
So focusing, for example, I'm doing this by age groups, focusing on people amongst 70 years and older, the global burden of disease tells me that amongst all people, 70 and up that have chronic kidney disease, about 55% of them should be female, are female in the population. And then what I can do is compare that with what share of what I see in BSBY which has these blue bars below. So for the same condition with people 70 years and above that, amongst hospital visits for chronic kidney disease, amongst people in that age group, about 30% or for females in BSBY right? And so, I mean, what the [GVD 00:15:46] prevalence sort of is telling me is, "If women were treated this condition on having chronic kidney disease, if women were treated the same way men were, what should the utilization, the female share of BSBY utilization for that age group look like?"
And so comparing it with BSBY tells me very clearly that this is not about differences in illness prevalence. In fact, it's that women are not being treated to the same extent that men are in BSBY. And we do this is we do this for, I think that's seven different conditions where we can map conditions in BSBY to those in GVD. And pretty systematic almost across the board, we find that in fact for, women or females, I should say, are more likely to be sick, have higher levels of sickness than males. And sometimes, the differences between what we see in BSBY and in the GVD is about 10 percentage points higher. So you can't, I think maybe not get to see that in my slide, but what we can do is we sort of essentially using these numbers, we estimate that there are about two [inaudible 00:16:58] missing female visits across just four of the top specialties in the three years that we study. Right? So women are systematically missing from this BSBY program.
Okay. So far what I've shown you is differences in the likelihood of coming in for utilization of BSBY, right. But what we also actually find is substantial gender disparities and the types of care seeking or care seeking patterns. Right? So for example, here in the light blue bars is the female share of all hospital visits in the public sector. And then the dark blue bar is shared hospital visits in the private sector. And the patterns broadly mimic what you saw earlier, even in public hospitals, women are most likely to be, women are smaller share of all public hospital visits than men are. But what you see is that these gaps are bigger in the dark blue bars, right? That women are even less likely to be observed in private care than they are in public care. And as I mentioned earlier, and it's sort of widely known, private care is typically perceived to be more expensive and better.
We also find this that the gender gaps are disparities on larger for higher value, more complex care relative to simpler services. Okay. And so another thing you might see as well, maybe the program wasn't super, it wasn't super well implemented right away, or people didn't really know about it, that all these things and that as things improve over time, the female share will go up. So here, the light blue bars is the number, pure number times, the number of visits in BSBY overtime 2019, since we only have data until October, it's not a complete year. And what you see is that yeah, the program is expanding. People are using it more and more, the levels of utilization are increasing pretty substantially year on year. What the black dashed line shows you is a female share of all of these visits, right?
And that this corresponds to the y-axis on the right hand side. And what you see is, female share is about 47, a little bit... About 47% in 2016. And it doesn't actually increase over time if anything, it decreases to about 44% by 2019. Right? So this really sort of goes against this idea that as the program expands, the female females are more likely to use it. In fact, we're seeing sort of be the opposite. And what this ends up meaning is that the spending this large amount of public spending on this program ends up being effectively male biased, right? So the gray dashed line is also sort of corresponds to the y-axis on the right side is the female shareable program spending. And it starts out at about 42% and drops to just under 40%. Overall, 57% of BSBY spending is on males. And to give you some benchmarks in richer, more developed countries, programs like this typically end up spending more on females than males for, because women either are sicker or they live longer. Right?
So then the question, I've eliminated a couple of a few immediately obvious explanations for these disparities, but what else could explain them? I classify these explanations broadly into sort of demand side and supply side. On the men side the first one explanation is that households are simply willing to spend less on female health, right? If this may be, there's sort of these economic arguments that it's rational, that the returns to female health for the household are lower than returns to investing in male health either because, females are less likely to participate in the labor force so they aren't being contributing to household earnings, or for example, because they don't stay with the family after marriage, they don't support the household in old age, et cetera. There's lots of this literature, or it could just be that households literally valued female health less than they do in what we would say now in mechanics, like taste-based discrimination. A second sort of possibility that comes up a lot in the qualitative literature actually, is that the female costs of care seeking are higher than for male.
So this is not a bias thing. It's just that it's more expensive for the household to take a woman or girl to the hospital, for the woman to go to the hospital. And so that's part of why female legalization is lower it the kinds of varies. So for example, women can't travel to the hospital alone or the safety concerns, they have to take a second person so that becomes more expensive, these kinds of things. And then the third thing is that, women actually just demand less care. They don't report their illness, they are less likely to say they need to go to the doctor, and I mean, this has also been [inaudible 00:21:55] them for them in a way this is sort of internalizing bias, but it's a slightly different thing from the household's bias. On the supply side instead, you might have the hospital or doctor discrimination, right?
It's possible that hospitals turn women away or mistreat them, or those are possible, but primary care doctors would be the first person that the visit a woman visits, doesn't take her illness seriously, and doesn't refer her up to the hospital right? Now let's look at these sort of evidence to figure out which of these is going on. Starting with this set of both the costs of care-seeking higher for women or women demanding less care.
This may be true for adult women, but it can't really explain the fact that we see some of the largest disparities amongst children, right? That like, because a child is likely to be taken by someone else in the household, the gender of the child, doesn't determine whether if it's the father or the mother, whoever it is. The gender of the child, doesn't determine the cost of care seeking for that child. Right? So the sort of barriers would be faced by an adult woman going to seek care for herself maybe. And similarly, women may be less likely to say that they were sick and things like that, but it's unlikely that that's the case for girls, right? So it's not that I'm not trying to say that these other factors are not part of the story, but that they can't fully explain the patterns we see. Turning to the supply side, one thing is that the private providers have an incentive to maximize patient volumes. So they really should be trying to do what they can to attract patients.
And instead, actually we see some larger differences in the private sector. But the other thing is that, it's hard for provider side bias to explain the fact that we see differences in the type of care seeking, right? It's hard for the provider to explain the fact that we see households less likely to seek care in the private sector for women than in the public sector. Turning to this last thing, what we do find instead is that there is evidence of gender bias in household resource allocation, which is consistent with this first sort of class of explanations. And again, to be clear, I'm not saying the others aren't part of it, but this is a major contributor. Okay. How do I make this single? It's like systematic. Okay.
Okay. I'm sorry, but this is sort of obscuring my screen a little bit. So the first thing, one of the things we did when we started this project was we conducted surveys with patients soon after they visited the hospital. We conducted about 20,000 surveys across a range of patients that received all kinds of services under BSBY. And we find that actually, although the program entitles patients to free care, in practice, it is not. Maybe if I just click on this and make it yeah. Okay. I'll have to address that later. So what we find is that in fact, hospitals do charge patients out of pocket for care that should be free. And this is widespread across services and patients. And what this graph is actually showing is... What we're trying to look at is what do these out of pocket what are these out-of-pocket charges do for gender disparities?
What I've plotted on the x-axis is the average amount that a hospital charges for its patients. And we are only using data on charges from new patients, so that it doesn't bias what we're doing, but we look at female utilization and then on the y-axis is the female share of visits at that hospital. And each of these dots is a hospital. And what you see essentially is there's a sort of strong, downward slope. Essentially, what this means is that the more a hospital charges on average, the less, the lower the female share of BSBY visits to that hospital or the less likely that households are taking girls to that hospital. And because everything we do in shares, what this basically means is that these costs that the hospital, these out-of-pocket charges that the hospitals are making disproportionately deter female care. They may be lowering care for males as well, but because we're looking at shares, we know that this is disproportionately affecting female care.
So this is taking sort of another cut from a different angle at the same question. So then there are these hospital out of pocket charges, but even if hospitals, don't charge anything, there are other costs of care seeking. One of which the travel cost, right? Distances, it's a decent proxy for the cost of care seeking mental hospital. So what I've shown here is on the x-axis is the distance from a village to the nearest hospital in BSBY in kilometers. And on the x-axis is the female share of BSBY visits from that village, right? And I've split it out by children in blue at the bottom, elderly in green in the middle, and the women of childbearing age. And the first thing you should notice is that, at every distance female, amongst children, female are only about 35% at max of all hospital visits from a village.
So this is sort of consistent with that first graph that I showed you just overall amongst children. There are these very, very large gender disparities. But the other thing, the point that I'm actually trying to make with this graph is that you see this like downward sloping, these curves are downward sloping. But essentially the greater the distance from a village to the nearest hospital, which we're thinking of it is that, the greater the travel cost of using BSBY from that village, the lower the female share of BSBY village visits from that village.
So just like with the previous graph where we were doing out out-of-pocket expenses here, sort of we can conclude that distance costs disproportionately deter female care. And we can do this in sort of progression for them, most of what I show you in the [inaudible 00:28:15]. Today is sort of pictures but when we find this, as I've shown you at all ages, we also find this with household fixed effects, which essentially means that we're saying even, within the same household, they're likely to travel, the distance dissuades female utilization more than it does male utilization. Okay.
So taking all of this kind of together, what we kind of conclude is, okay, so the basic intuition is that, if a household is gender bias, it's going to be willing to allocate fewer resources to female than to male care, right? Right. That's the bias resource allocation. What I then shown you is that BSBY does not fully eliminate the cost of care seeking. A, there are the fact that hospitals are charging patients out of pocket, but even if they'll have government makes sure that hospitals are not charging patients out of pocket, there are these other costs like travel for long [inaudible 00:29:11], et cetera. Because of the gender bias, these costs of care seeking, detour care seeking for females more than they do for males. And that's what sort of results in gender disparities.
So then the natural question is, does reducing the cost of care seeking reduce gender disparity? And so to do this, what we take advantage of the same intuition of the distance graphs that I showed you earlier. What we got to do is in early 2018, the government impaneled a whole bunch of new hospitals. And impaneling a hospital near a village that didn't previously have a hospital close by, is a way of sort of suddenly reducing the cost of care seeking for people from that village. Right? So what I do is I consider a bunch of villages that suddenly had a hospital impaneled in 2018 and consider those the sort of treated villages and compare them with a bunch of villages that did not... These are all villages that previously did not have a hospital, right?
And some of them got one in 2018, these got the treatment and others did not. And what I can do is sort of compare the BSBY utilization for males and females across these two types of villages before one of them got the treatment and they should look sort of similar. And then once the ones that got the treatment that got this hospital impaneled nearby, I should see a change increase in BSBY utilization because the cost of using BSBY, from that village decrease. Okay. So I can do this by males and females and look at what this [inaudible 00:30:48] disparity.
And so what this is doing is essentially showing an event study [inaudible 00:30:56] what I just talked about, focus on the red diamonds, which are the female patients. And so the red vertical line is the time date of the impanelment for the treatment group, the date when they got a hospital nearby within 20 kilometers. And so what you see is, in the months before the impanelment, the treated and untreated villages have, the female levels of utilization were very similar. Sorry the outcome here is the number of [inaudible 00:31:24]. And then this looks very similar across these two groups of villages, but once the treated group villages get a hospital nearby, the female levels of utilization increased significantly and substantially and sort of immediately. But now look at the blue dots, which is for the same sort of thing, but for male visits. And what you see as a male visits also increased.
So when a hospital comes nearby a village, it gets cheaper households start bringing in their women more, but they also start bringing in their men more. And the effects from them are very similar to those for women. And so what does this do for female share sort of the disparity, the gap between men and women? And sort of showing the scene of that study here, but with female shares the outcome. And what you see is essentially female share does not go down at all. Okay. And so what this means is, I mean, I've shown you earlier that care seeking costs, disproportionately deter female utilization and contribute given gender disparities because of the bias in the household. What I showed you here is decreasing the cost of care, which I proxied with closing the distance a bit increases female levels of utilization.
It makes sense, right? You'd make it a little bit cheaper. Households are more likely to bring in their females. However, it doesn't necessarily reduce gender disparities because males benefit as much or could benefit in some cases more than females and sort of in econ speak, the marginal beneficiary is male. Okay. And I'm not going to get into sort of what this is, but it sort of has to do with sort of the way the demand curves look for male and female care. But these sort of intuition, as you think about it, like when you introduce a little bit of a subsidy, some women might come in, but the first people to benefit from the subsidy are going to be male. You reduce it further, and for a while, those sort of primary people to benefit from the subsidy are going to be male.
And you really have to get to a much higher level of subsidy where your households are facing. I don't know exactly what that point is, but it's going to be much closer to patient zero costs before you get to the point where the households, and now also the men have all kind of benefited already, and now the households are also starting to bring [inaudible 00:33:39]. And to sort of put it in econ language, get to the point where the marginal beneficiary is female. Okay. So that's sort of the big intuition. The last part of the paper is, looking at this, I've sort of shown you what happens like the relationship between the cost of care seeking that a household faces and subsidies reducing that, and what that does to gender disparities. A different take on this is what if instead you change, you sort of tried to strengthen position of females and does that rather than focusing on the subsidy, so to change, change the way households, think about peanuts and households, right?
And what does that do for gender disparity? To study this I'm just going to do this very quickly in one slide, but we look at the long-term exposure of households to female political leaders. So as a way of seeing what happens when you change the position of [inaudible 00:34:38]. India has this system of Gram Panchayat, which of these village level elected councils that are responsible for providing local public services, like certain health care services, sanitation, drinking, water, things like this, right? For a couple of decades now there's been a policy where one third of the head positions of these elected councils or Sarpanch positions are reserved for females. And these one third of the villages are selected randomly in each electoral term, and it's randomly with replacements. So the same village could potentially get selected the second time.
And what we confirmed that, in the absence of these reservations, almost no women, no Sarpanches are filled by women, but their actual compliance with the reservation is actually fairly high. And so the reservation policy like induces, it substantially changes the likelihood of exposure to a female Sarpanch. And because the selection of villages was random, you can treat this like an RCT and compare villages that didn't get these reservations. And what we do is we sort of link our BSBY data to three electoral terms of reservations data. So each of these terms is five years. So where essentially we're looking at those without no reservation, since your exposure and up to 15 years of exposure to a female Sarpanch. And prior studies have actually looked at this and have found that these reservations in these villages that have these female leaders, gender attitudes change, and that households make more investments in girls.
So it does seem there's some prior evidence that it changes the way households perceive women and invest in girls. Okay. What we find very quickly is that in fact, exposure to a female Sarpanch does in fact, increase the team share or BSBY villages from that village. However, the effects are very, very small, and we find that they only actually show up after at least two electoral terms of exposure. And this is actually consistent with other studies that also find that some of these effects take a while to show up, which is sort of consistent with this norm changing, takes awhile. The other thing is that we don't find that elderly women benefit from this. The positive effects, are all among children and childbearing age women. And then we conduct surveys both with households and with Sarpanches to kind of dig into the mechanisms a little bit.
And what we find is that females Sarpanches are more likely to invest in maternal and child health. And what would the [ushers 00:37:15] and this kind of thing. We also find some evidence that the female agency in these villages may increase. And that sort of key takeaway from this is that Gram panchayat reservation had nothing to do with BSBY. But what we find is that, policies to strengthen the position of women can have complimentary downstream effects on the extent to which female benefit from other programs, which is sort of encouraging and actually somewhat surprising in this context. However, changing attitudes and these kinds of processes are sort of slow and incremental. And we can't wait around for this to take in order to make sure that women and girls benefit from BSBY as much as boys and men do. We can't wait decades for this kind of progress.
And so this is my final slide and taking everything sort of that we have found together. And I think it's fair to say that many of the findings from here sort of the theoretical implications probably extend to social programs besides BSBY. But essentially the cost of using social programs, exacerbate gender disparities in the presence of gender bias. Reducing the cost of utilization is likely to increase the levels of utilization so we should do that. More women are going to benefit more women that use BSBY when the cost was reduced. So it's a worthwhile thing. However what we've sort of shown is that in the presence of gender bias, because these past reductions are gender mutual, they affect everybody. They may not reduce gender gaps because males may benefit as much or more as females until you need to get to a very high level of subsidy.
It's not a foregone conclusion, which is actually how people usually think. It's like, "Oh, you make it cheaper it's going to sort of solve the inequality problem." And we find that that's not the case. And sort of in conclusion and somewhat not surprising when you think about it, reducing gender disparities is going to require more than sort of just universal policies but in fact, gender targeted interventions that are either reducing female specific barriers to utilization of these programs and in the long run that are strengthening position of women and how we value them in society. Thank you very much. That's all I have.
Nafiz Hassan:
Thank you so much. It's a really, really fascinating deep dive and really, I think the material that you've shown us here is so hard to find in a general sense. So I think it really raises really interesting questions to think about. So we have a bunch of questions and I'm going to call upon our audience to ask them. Can I start with [Silva 00:40:01] please? Because I think they had a clarifying question from one of your earliest slides. Silva?
Silva:
Good morning and good evening. This is Silva. My quick clarification question about the share of spending on female patients. Does it mean the share of claims or are you referring to the budget spending? This was my question, actually.
Radhika Jain:
Yeah, so actually the blue bar chart, where is the share of claims, that's the share of hospital visits. But the share of spending was actually the amount that the government is reimbursing out, spending on the program in terms of reimbursements to hospitals, the value of all of it.Yeah
Silva:
Basically the value of the claim, amount claimed?
Radhika Jain:
Yeah.
Silva:
Thank you. Thank you. The other quick thing to check the system, and I don't know that I can add it to this where you say that it is not really reducing the disparities. Mainly equally consumer increase is equal and for both. So let's make a male and female. I wonder, particularly in situations like Roger, Stan, probably we have not reached a breaking point. So the basic utilization, and then any additional services available may get utilized differently because now everyone is under utilizing or not getting adequate services. So any additional service come, people will compete and then get used more or less equally. So I wonder if they're ever reached a breaking point. And then if you look at property, we'll be able to capture that differences. Thank you.
Radhika Jain:
Sure. I hope I'm understanding this correctly. I mean, I think don't quite get the question. But I think what we are seeing essentially is the gender differences across all kinds of services and these are essential services, right? So for example, when I talked about chronic kidney disease, that's dialysis care, much of that is dialysis care and that's, things that if you don't get dialysis, you die, right? If you don't get it within a few weeks. So I think the disparities it's true that this is not primary care, but the disparities that we are seeing right now are already in a range of essential hospital services.
Nafiz Hassan:
Thank you. Thank you. We have a question from [Manya Gupta 00:42:41]. Manya would you like to ask a question?
Manya Gupta:
Yeah. Hi. I wanted to ask about whether the Sarpanch effects [inaudible 00:42:50] you change or through increased investment and policy changes from these Sarpanches. And if there's any way to measure, which one of the two might be a larger source of the effect. Thank you.
Radhika Jain:
Sure. It's a good question. And I went through this Sarpanch results fairly quickly, but sort of, what we did, we did do surveys with the Sarpanches, male and female Sarpanches to understand what their priorities are, what they spend on the same thing. And we also did surveys with households in these villages that were and were not exposed to measure their attitudes, as well as the care seeking behavior and, female agency and this kind of thing. What we find and I can't quantify which of these aspects is going to contribute, how much each of these contributes. But what we find is [inaudible 00:43:38].
Nafiz Hassan:
Can you please keep your mics on mute? Thank you.
Radhika Jain:
What we do find is... Sorry, I lost my train of thought for a second. So we do find strong evidence, clear evidence that females Sarpanch are much more involved in health care activities and invest more of their time in this. They meet with the ushers, they do more to encourage women and girls to come in to get maternal health services. So there's definitely evidence that female Sarpanches are investing more in health. But we, we don't see that they're directly helping women go use BSBY. Instead of these like longer term, just general prioritization of health and specifically the health of women and girls. In terms of attitudes and agency, we don't see a lot of change in attitudes, but I will say that, we didn't have the ability to measure this in great detail.
I don't, I wouldn't be surprised, I wouldn't rule it out. What we do find some evidence of is some change in agency of women that they can... They're a little bit more able to leave the village, or are a bit more involved in household decision-making, this kind of thing.
But in practice, I think all of these sort of mechanisms are [inaudible 00:45:06]. What we tried to show in the paper is that the things that I've mentioned, plus the fact that you don't see Sarpanch exposure having an effect right away. It's not that these Sarpanches are just helping women go [inaudible 00:45:22]. Well, these effects are taking decades to unfold. And so it's just all more consistent with a sort of these like longer term sort of mechanisms, both investments in health and changes in the agency.
Nafiz Hassan:
Thank you so much. Lots more questions pouring in. So can I ask everyone to keep it to one question. I'm going to ask [Mikil 00:45:52] [inaudible 00:45:51] to ask his question.
Mikil:
Hi Radhika.
Radhika Jain:
Hi.
Mikil:
I have a question on your section two. And we have showed that when this new hospital gets impanel, utilization goes up. Is it that a physically new hospital was built or was it that an existing hospital was empaneled? Basically I'm wondering, is that the case that people were always seeking care at hospital and then suddenly it becomes a panel so that's why you're seeing that BSBY claims coming in? Or is it that they built a BSBY hospital, where there previously was none. And if that's the case, do you think it's the BSBY effect or just there's a new hospital [inaudible 00:46:27] effect?
Radhika Jain:
Yeah. No, it's a good question. Let me try to address this carefully. So we cannot see whether hospitals... I don't have detail on things outside BSBY. But I think in a lot of cases, I don't think BSBY cause all of these hospitals to start from nowhere. I think a lot of cases, these hospitals were there and started choosing to impanel in BSBY or where the government approved their impanelment over time. And so then what does this mean for... So it's possible that to some extent, the patients were getting some care outside BSBY anyway. But regardless A, this should be a reduction in the cost because to some extent that hospitals are getting subsidized in a way that they weren't before, right now to BSBY. And so it would be very odd to think that doesn't have any effect on utilization.
But part of what we're trying to get at here is like, it's not saying... Another side by the way is also that we actually... No maybe that's not relevant. But what we trying to say here is what does reducing the costs within the program do to gender disparities of utilization in the program, right? And so not necessarily sort of gender disparities and utilization overall. And so the thing that I was just about to say, I think is relevant. I didn't show it today, but what is actually slightly disturbing is that, I can overall be used NSS data to look at female share of hospitalization before and after BSBY and we see almost no change in fact. So overall as well, it doesn't actually seem like BSBY is improving the female share hospitalization.
Nafiz Hassan:
Thank you. Can I ask [Mary Ranger 00:48:27] to ask a question?
Mary Ranger:
Yeah. Hello. It's a very interesting presentation that you got there Radhika. So my question... It's mostly very general. So it's basically regarding the health seeking behavior of the people of that population before and after the program policy. And the other one is also with regards to the decision-making process that we are seeing. Because mostly what we really find in the Indian population that the woman, the decision making process is mostly with regards to the family or the husband of the family. So I would just like to know on that. Thank you.
Radhika Jain:
About decision making, about healthcare seeking and utilization of BSBY you mean?
Mary Ranger:
Yes, yes.
Radhika Jain:
Yeah. I mean, we also find some evidence that for sure, a lot of the decisions around spending and CASI [inaudible 00:49:29]. Even though typically it's the female sort of mother or females head of the household, that's involved in care provision for children. It's usually the man who is much more involved in decision-making. Women of all the different kinds of decisions, women are more involved in health decisions and other types of things, but even so and I guess we're not making this, that could mean that a lot of this bias that we're seeing is actually just the man in the household that is biased. And I can't say very much about that it's possible, or it's possible that both of them men sort of seek providers. The man and his wife let's say are making this decision together it's possible. But yes, I think that in a lot of cases, these decisions are being made by the males in the household about whether to use BSBY or not.
Nafiz Hassan:
Thank you so much. Can I ask [Nikhil Panti 00:50:27] to ask one of his many questions and then I can call you back if we have time?
Nikhil Panti:
Sure. Thank you. Thanks Dr. Jain, for your detailed and insightful presentation. I'm going to plug two of my questions together because it's too provocative. I wonder to what extent is cost as discrimination, segregation, implicit or explicit bias also part of the story of gender bias that you're telling and your study account forecast in any way and connected with that, to what extent is women's labor dependent on their costs or on structural factors, also part of one of the reasons or lower as outcomes among women?
Radhika Jain:
Okay. So on cost, I mean, I would love to study that in part because cost and gender dynamics intersect in very interesting ways in India in ways that... But the strength of this study is using the large data set that we have from the administrative claims data, and that does not include cost. And in fact, we thought about trying to sort of classify the names, but a lot of times the names that are recorded are only the first name, so it doesn't allow us to look at CASI, so I can't actually tell you a whole lot about past dynamics in the utilization of this program. It is something actually that I want to study in the future. Just looking at sort of geographic disparities by where lower class are more prevalent.
I don't know if with the labor question, I don't know if you meant that, is it the women's labor itself that's causing health problems or whether you mean that what I touched on, which is that because women's labor force participation is low, they don't contribute to the household income. And that's why there's less utilization for them. The former, I think it's possibly part of it. I think actually a lot of the biases, I should just [inaudible 00:52:33] from discrimination, households don't value the health of women as much as men. I can't say very much about whether, if it's the other thing you meant, I don't really know very much about what the role of the labor of women, has on their health. Sorry, in this context. I don't know.
Nafiz Hassan:
Can I ask [Bhavna 00:52:54] to ask a question, please?
Bhavna:
Yes. Good evening everyone. My question was regarding the cost of treatment basically the cost of treatment contributing to gender bias. So we're focusing on mode of public sector, hospitals, which are well equipped and which are maintained properly, help in this situation because they can actually reduce the cost of which people have to pay.
Radhika Jain:
Yeah, yeah. I think, I mean, it's a big... For that to really work, it's going to need to do more than just reduce the cost. Right? You also have to improve the quality at these public hospitals. It's another way of saying sort of the broader point I was trying to make is that reducing the cost can help, but you really have to get down to very, very low costs. And what we have found actually in our data is actually the public hospitals charge quite a bit as well.
It's lower than what private hospitals charge, but they're also judging patients and they're not providing very good care. So if that is going to be a part of the strategy... If you think about it, India had for decades focused on trying to provide cheap healthcare through its public hospital network. And it has achieved some gains, but it's far from closing gender disparity. So I think it can be part of the solution, but you're it's going to require a lot more investment in strengthening and making and monitoring these public hospitals, making sure they're open easy to access high quality and actually don't charge patients anything, and that may help some.
Nafiz Hassan:
Thank you. Tariq, would you like to ask your question now?
Tariq Thachil:
Yeah. Thanks. Radhika thank you so much for this amazing talk. It's really insightful. The data that you have is phenomenal adventure through some of this landscape. So I had one kind of larger question about utilization. Like, is there evidence of BSBY evidence executed. Where is it drawing its patient pool from? So was it coming at the expense of public hospital visits or over kind of private, non MBBS or low cost private alternatives, just given that that's such a prevalent use of practicing of private sector kind of medical visits in India.
And so I was just curious about whether you have, it's a little bit separate from the focus of your talk, but just kind of where patients are coming from in accessing the BSBY program in private hospitals. And then the kind of question more on kind of what you approving is, I also have this thought about failure and the kind of risks, even with utilization, you're kind of getting at that with the out-of-pocket expenses. But it seems to me like you're with the out of pocket expenses, the premise is that a BSBY visit is always successful.
And whether that was true or whether there are cases where claims fail. And in particular, I was wondering if men are often going to be the first point of call with such a scheme, and if there's experience and success versus experience of failure, that may then determine whether women are likely to be able to utilize that. And so whether you see kind of within a household learning effects, I mean, I know you did household fixed effects, which you're looking at slightly differently, but if you have multiple claims within a household, is there any way of easing out how an initial male experience with BSBY informs the likelihood of a subsequent female visit?
Radhika Jain:
Okay, great. Okay. So let me try to get that second one first. If we had a denominator, if we have a number of people enrolled, then I could actually and I would know it by household, then I could actually look at household utilization, right? The problem now with our data, is it really makes it very difficult for us to look at whether, a male's utilization first leads to subsequent female utilization, because I don't know, maybe there's just those households don't females as an option. But I think overall it's absolute... I think it's very likely, I think this has been documented in other studies of health insurance programs and any programs in India, actually that bad... People face all sorts of hurdles in utilizing them at public expenses. Just one of them might be turned away by the hospital. Husbands don't have so much incentive. Private hospitals don't have a huge incentive to turn people away because they get money from it, but almost certainly those kinds of problems deter, dampen care seeking down the line. And maybe I like the idea and may do some more for females.
Tariq Thachil:
But like, I don't know if during the surveys or whatever you got a sense of. I mean, was there ever a situation in which a BSBY claim is not actually on or a payment is not made through that? I mean, is it really pretty much automatic, that you enroll and make a claim on that, the payment is processed>
Radhika Jain:
Yes. Because that's done between the insurer and the hospital. So the households, it's not a sort of reimbursement, which is one of the things they did to try to reduce the barriers, to care seeking in this program. They try to make enrollment automatic and reimbursement is, the households literally should swipe their card and the hospital gets reimbursed. That's said, if the hospital has problems, we don't actually find too much problem initially in the reimbursement. But if the hospital has problems, then it may contribute to what it starts charging patients, because it's anticipating not getting paid by the government or something, but I can't really see that. On the private and where these patients are going to come from... I don't remember the public private mix from before and after. It's a bit complicated because the sort of services BSBY would cover different [inaudible 00:58:43] changed.
I wish I should've had this number in my head, but my sense is that, the program did increase utilization of the private sector. And some of that is coming from previously public sector utilization. And because we see increasing private sector utilization and not that much increase of public sector utilization over time. And so some of that is drawing people away from the public sector, but I think a bunch of it is actually joining people from... It's either subsidizing people that were getting care already, right? So it's not necessarily inducing all new care seeking, but it's making it much cheaper for them. And to some extent, joining in new patients. It's kind of hard to get great data on that. The best we have is NSS, and it's not quite comparable, but that's my sense of what's going on.
Nafiz Hassan:
Thank you. I think we have time for one last question and I'm going back to the Nikhil, because he had a question on methods. Nikhil, do you want to ask that?
Nikhil Panti:
Sure thank Nafiz. Dr. Jain I was curious about the patient surveys that you mentioned as part of your methodology. And I was just curious about what they entail. Were they qualitative in any sense? Did they allow you to probe at the questions and the variables in a way that was perhaps different to the larger quantitative dimension. Thank you.
Radhika Jain:
Sure. I didn't have time to go into all of these details, but we use a few different types of... This is all sort of part of a bigger project looking at health insurance program. To do the surveys, because we didn't have a huge budget, we use the phone numbers in the administrative claims data to conduct phone surveys with patients. And so we could see, and we would get these claim data every two weeks. We could see people who had just visited BSBY hospital for a service and we could call them... We tried to do this within two or three weeks of their claim to find out more details about their visit. And because we were using these surveys for various purposes, I mean, these were mostly quite large scale.
I told you that when we were measuring out-of-pocket spending, that was, the sample size of about 20,000 patient surveys. Right? And those were largely sort of quantitative surveys. The information on the surveys with Sarpanches as well as some of the surveys we did to try to understand the attitudes included some more open-ended questions to get at what was going on, how people were interacting with the Sarpanche, but I will also say I'm not a qualitative methods expert, so we didn't do this in some big systematic way. And some of the things that I'm telling you about the mechanisms about how the steam of exposure to Sarpanches worked was, is partly from sort of open-ended interviews to understand a little bit combined with mos of it is really from sort of more quantitative surveys.
Nafiz Hassan:
All right. Thank you so much. So we'd come to the end of the hour. Thank you Dr. Jain, for such a wonderful presentation and for sparking so many questions. Thank you audience for attending this once again. Do remember that next week we have Dr. Paul Novosad from Dartmouth College. So if you're interested in that, please sign up on the CASI website and see you all next week. Bye.
Radhika Jain:
Thank you so much.